Add AVX512 support in ATen & remove AVX support (#61903)
Summary:
Pull Request resolved: https://github.com/pytorch/pytorch/pull/61903
### Remaining Tasks
- [ ] Collate results of benchmarks on two Intel Xeon machines (with & without CUDA, to check if CPU throttling causes issues with GPUs) - make graphs, including Roofline model plots (Intel Advisor can't make them with libgomp, though, but with Intel OpenMP).
### Summary
1. This draft PR produces binaries with with 3 types of ATen kernels - default, AVX2, AVX512 . Using the environment variable `ATEN_AVX512_256=TRUE` also results in 3 types of kernels, but the compiler can use 32 ymm registers for AVX2, instead of the default 16. ATen kernels for `CPU_CAPABILITY_AVX` have been removed.
2. `nansum` is not using AVX512 kernel right now, as it has poorer accuracy for Float16, than does AVX2 or DEFAULT, whose respective accuracies aren't very good either (#59415).
It was more convenient to disable AVX512 dispatch for all dtypes of `nansum` for now.
3. On Windows , ATen Quantized AVX512 kernels are not being used, as quantization tests are flaky. If `--continue-through-failure` is used, then `test_compare_model_outputs_functional_static` fails. But if this test is skipped, `test_compare_model_outputs_conv_static` fails. If both these tests are skipped, then a third one fails. These are hard to debug right now due to not having access to a Windows machine with AVX512 support, so it was more convenient to disable AVX512 dispatch of all ATen Quantized kernels on Windows for now.
4. One test is currently being skipped -
[test_lstm` in `quantization.bc](https://github.com/pytorch/pytorch/issues/59098) - It fails only on Cascade Lake machines, irrespective of the `ATEN_CPU_CAPABILITY` used, because FBGEMM uses `AVX512_VNNI` on machines that support it. The value of `reduce_range` should be used as `False` on such machines.
The list of the changes is at https://gist.github.com/imaginary-person/4b4fda660534f0493bf9573d511a878d.
Credits to ezyang for proposing `AVX512_256` - these use AVX2 intrinsics but benefit from 32 registers, instead of the 16 ymm registers that AVX2 uses.
Credits to limo1996 for the initial proposal, and for optimizing `hsub_pd` & `hadd_pd`, which didn't have direct AVX512 equivalents, and are being used in some kernels. He also refactored `vec/functional.h` to remove duplicated code.
Credits to quickwritereader for helping fix 4 failing complex multiplication & division tests.
### Testing
1. `vec_test_all_types` was modified to test basic AVX512 support, as tests already existed for AVX2.
Only one test had to be modified, as it was hardcoded for AVX2.
2. `pytorch_linux_bionic_py3_8_gcc9_coverage_test1` & `pytorch_linux_bionic_py3_8_gcc9_coverage_test2` are now using `linux.2xlarge` instances, as they support AVX512. They were used for testing AVX512 kernels, as AVX512 kernels are being used by default in both of the CI checks. Windows CI checks had already been using machines with AVX512 support.
### Would the downclocking caused by AVX512 pose an issue?
I think it's important to note that AVX2 causes downclocking as well, and the additional downclocking caused by AVX512 may not hamper performance on some Skylake machines & beyond, because of the double vector-size. I think that [this post with verifiable references is a must-read](https://community.intel.com/t5/Software-Tuning-Performance/Unexpected-power-vs-cores-profile-for-MKL-kernels-on-modern-Xeon/m-p/1133869/highlight/true#M6450). Also, AVX512 would _probably not_ hurt performance on a high-end machine, [but measurements are recommended](https://lemire.me/blog/2018/09/07/avx-512-when-and-how-to-use-these-new-instructions/). In case it does, `ATEN_AVX512_256=TRUE` can be used for building PyTorch, as AVX2 can then use 32 ymm registers instead of the default 16. [FBGEMM uses `AVX512_256` only on Xeon D processors](https://github.com/pytorch/FBGEMM/pull/209), which are said to have poor AVX512 performance.
This [official data](https://www.intel.com/content/dam/www/public/us/en/documents/specification-updates/xeon-scalable-spec-update.pdf) is for the Intel Skylake family, and the first link helps understand its significance. Cascade Lake & Ice Lake SP Xeon processors are said to be even better when it comes to AVX512 performance.
Here is the corresponding data for [Cascade Lake](https://cdrdv2.intel.com/v1/dl/getContent/338848) -
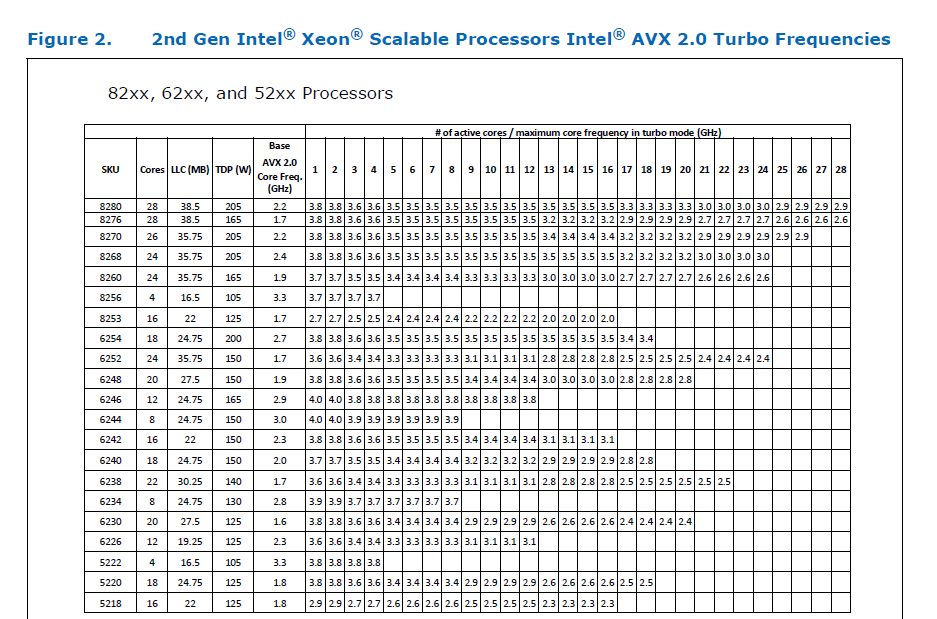
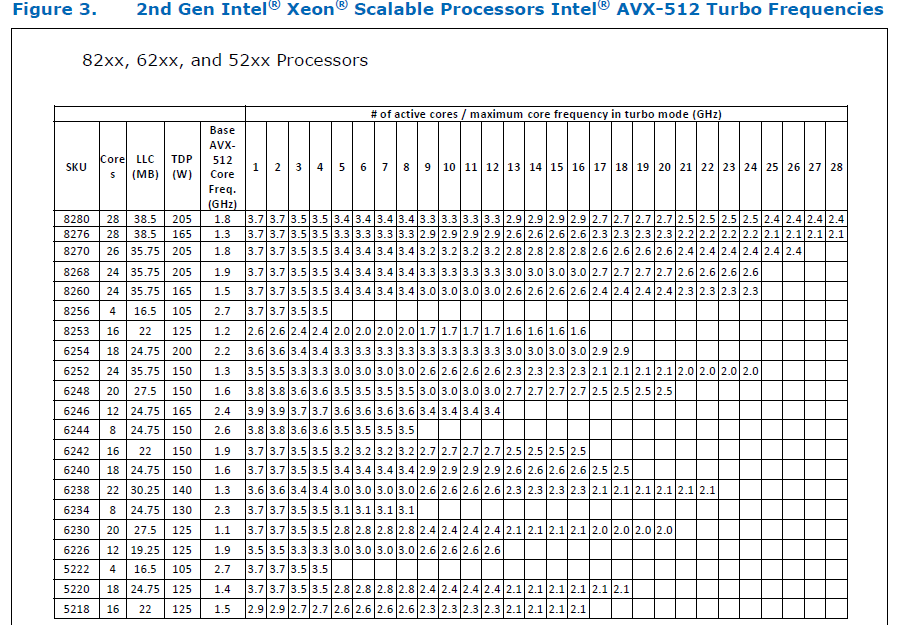
The corresponding data isn't publicly available for Intel Xeon SP 3rd gen (Ice Lake SP), but [Intel mentioned that the 3rd gen has frequency improvements pertaining to AVX512](https://newsroom.intel.com/wp-content/uploads/sites/11/2021/04/3rd-Gen-Intel-Xeon-Scalable-Platform-Press-Presentation-281884.pdf). Ice Lake SP machines also have 48 KB L1D caches, so that's another reason for AVX512 performance to be better on them.
### Is PyTorch always faster with AVX512?
No, but then PyTorch is not always faster with AVX2 either. Please refer to #60202. The benefit from vectorization is apparent with with small tensors that fit in caches or in kernels that are more compute heavy. For instance, AVX512 or AVX2 would yield no benefit for adding two 64 MB tensors, but adding two 1 MB tensors would do well with AVX2, and even more so with AVX512.
It seems that memory-bound computations, such as adding two 64 MB tensors can be slow with vectorization (depending upon the number of threads used), as the effects of downclocking can then be observed.
Original pull request: https://github.com/pytorch/pytorch/pull/56992
Reviewed By: soulitzer
Differential Revision: D29266289
Pulled By: ezyang
fbshipit-source-id: 2d5e8d1c2307252f22423bbc14f136c67c3e6184